V. Dilli Ganesh, T. J. Nandhini
1Department of Mechanical Engineering, Saveetha School of Engineering, Saveetha Institute of Medical and Technical Science – SIMATS, Saveetha University, Chennai, India
2Department of Computer Science and Engineering, Saveetha School of Engineering, Saveetha Institute of Medical and Technical Science – SIMATS, Saveetha University, Chennai, India.
*Author to whom correspondence should be addressed:
nandhinitj67@gmail.com (T. J. Nandhini)
ABSTRACT
This study focuses on predicting surface roughness in the turning process of Monel K-500 super alloy by employing simulated annealing and a Genetic Algorithm (GA), comparing their effectiveness with experimental analysis. The turning experiments were conducted using the L9 orthogonal array, and surface roughness values were measured for each combination of cutting parameters. The predicted surface roughness values from both simulated annealing and the Genetic Algorithm were then compared with the experimental measurements. The results revealed that both simulated annealing and the Genetic Algorithm effectively predicted surface roughness with high accuracy. The predicted values from both methods closely aligned with the experimental data, demonstrating their capability to optimize cutting parameters for an improved surface finish. Simulated annealing efficiently explored the parameter space and provided reasonably accurate predictions, validating its ability to optimize the turning process. Similarly, the Genetic Algorithm exhibited robust performance, converging to near-optimal solutions for minimizing surface roughness. The comparison between simulated annealing and the Genetic Algorithm revealed similar predictive accuracy, showcasing the efficacy of computational intelligence techniques for surface roughness prediction in the turning of Monel K-500 super alloy. These optimization methods hold significant potential in enhancing machining processes, enabling accurate selection of cutting parameters, and improving overall component quality. In conclusion, the successful application of simulated annealing and the Genetic Algorithm underscores their value as powerful tools in the manufacturing industry. These techniques contribute to the advancement of machining strategies and enhance product quality for challenging materials like Monel K-500, making them crucial for various engineering applications that require high precision and performance.
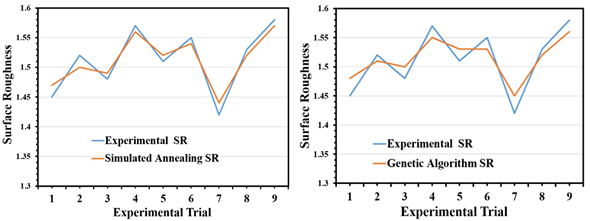
Significance of the study:
This study demonstrates the effectiveness of simulated annealing and genetic algorithms in predicting surface roughness during the turning of Monel K-500 super alloy, highlighting their potential to enhance machining processes. Accurate prediction and optimization of cutting parameters can significantly improve surface finish and component quality, making these computational intelligence techniques valuable for high-precision engineering applications.
Summary of the study:
This study demonstrates the effectiveness of simulated annealing and genetic algorithms in predicting surface roughness during the turning of Monel K-500 super alloy. Both methods closely aligned with experimental measurements, with average deviations of ±0.02 μm and ±0.03 μm, respectively. These techniques can optimize cutting parameters, improve surface finish, and enhance component quality, proving valuable for high-precision machining of challenging materials like Monel K-500.