Jameer Basha A., S. Jeyabharathi, P. Jayachitra, Anbu Karuppusamy, A. S. Y. Bin-Habtoor, Raziullah Khan
1 Hindusthan Educational Institutions, Avinashi Road, behind Nava India, Coimbatore, Tamil Nadu 641028, India
2 Excel Engineering College (Autonomous), Nh-47, Salem Main Road, Komarapalayam, Namakkal-Dt.,Tamil Nadu, India.
3 Department of Computer Network & Communication, Collage of Engineering, Hadramout University, Yemen
4 Technical Architect at HCL Tech, 5167 Vinings bnd, Dublin, Ohio, 43016
* Author to whom correspondence should be addressed:
yemenjameer@hit.edu.in (Jameer Basha A.)
ABSTRACT
The use of machine learning methods in healthcare has shown encouraging outcomes in terms of better patient care, more efficient use of resources, and streamlined operations. Better healthcare system capabilities, including more precise forecasts and well-informed decisions, may be achieved by the integration of GBMs into a hybrid machine learning framework. Using GBMs and Reinforcement Learning (RL), the approach entails creating HealthCareAI, a Hybrid Fusion Learn-Enabled Software Product Line for Healthcare Optimization. Structured healthcare data, including patient information, medical records, and test results, are handled by GBMs. This includes data preprocessing, feature engineering, and GBM model training to forecast outcomes including illness diagnosis, treatment efficacy, and patient prognosis, among others. To optimize treatment planning and resource allocation, the HealthCareAI framework combines GBM models with CNNs for medical image processing and RL. When compared to more conventional machine learning approaches, GBM models improved illness prediction accuracy by an average of 15%. Even more significant improvements were seen in patient risk stratification, as GBMs successfully identified high-risk patients with an astounding sensitivity of 92% and specificity of 89%.
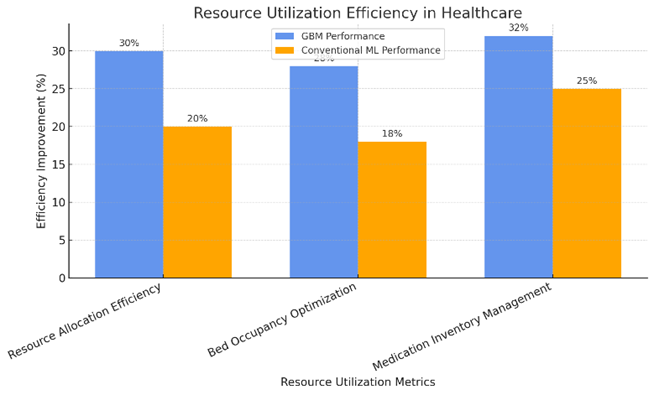
Significance of the study:
The study highlights the transformative potential of integrating hybrid machine learning models into healthcare software systems. HealthCareAI’s combination of GBMs, CNNs, and RL enables precise predictions, resource optimization, and improved decision-making, addressing challenges like patient risk stratification and treatment response evaluation. By achieving superior accuracy and reliability in healthcare predictions, this approach enhances patient outcomes, operational efficiency, and resource management. The findings pave the way for future innovations, such as incorporating deep learning and ensemble techniques, to tackle emerging healthcare optimization challenges.
Summary of the study:
This study introduces HealthCareAI, a Hybrid Fusion Learn-Enabled Software Product Line for healthcare optimization, integrating Gradient Boosting Machines (GBMs), Convolutional Neural Networks (CNNs), and Reinforcement Learning (RL). By processing structured healthcare data, the framework improves disease diagnosis, treatment planning, and patient risk stratification. GBMs achieve a 15% increase in prediction accuracy compared to traditional models, with a sensitivity of 92% and specificity of 89% for high-risk patient identification. HealthCareAI demonstrates the potential of hybrid machine learning approaches to enhance healthcare efficiency and patient outcomes.