Hakkem B., Mahendrakan K, Prabu S., Reyazur Rashid Irshad
1 Hindusthan Educational Institutions, Avinashi Road, behind Nava India, Coimbatore, Tamil Nadu 641028, India
2 Department of Electronics and Communication Engineering, Mahendra Institute of Technology, Namakkal, Tamil Nadu-637503, India
3 Department of Computer Science, College of Science and Arts, Sharurah 68341, Najran University, Saudi Arabia
* Author to whom correspondence should be addressed:
dr.mahendrakan.k@hit.edu.in (Mahendrakan K)
vsprabu4u@gmail.com (Prabu S)
ABSTRACT
Energy efficiency in Wireless Sensor Networks (WSNs) is a crucial challenge due to the limited power supply of sensor nodes. This paper introduces an AI-driven adaptive clustering mechanism to address this issue, enhancing energy efficiency and prolonging network lifespan. The proposed framework integrates machine learning (ML) techniques with bio-inspired optimization algorithms, such as Genetic Algorithm (GA) and Salp Swarm Optimization (SSO), for dynamic cluster head (CH) selection and adaptive clustering. Unlike traditional protocols like LEACH and HEED, which rely on static or random CH selection, our approach leverages real-time network conditions, node heterogeneity, and energy status to optimize clustering decisions. Simulation results reveal significant improvements in energy consumption, network stability, and scalability. The proposed method reduces total energy consumption by approximately 30% compared to LEACH and 20% compared to HEED. Furthermore, the network lifetime is extended by 25%, and data throughput is increased by 20%. This improvement is achieved through intelligent CH selection, which balances the energy load among nodes and prevents premature node failures. This study highlights the potential of AI-driven optimization for adaptive clustering in WSNs, making it suitable for energy-critical applications such as environmental monitoring, smart cities, and healthcare. By demonstrating the effectiveness of combining ML with bio-inspired algorithms, the proposed method provides a robust solution to the energy depletion challenges in WSNs. Future research will focus on incorporating reinforcement learning and multi-objective optimization to further enhance adaptability and address diverse performance metrics.
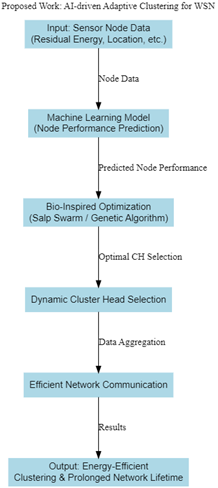
Significance of the Study:
The study addresses the critical challenge of energy efficiency in WSNs, leveraging AI-driven optimization to overcome limitations of traditional clustering protocols. By balancing energy load among sensor nodes, the proposed framework prevents premature failures, enhancing network scalability and sustainability. This innovation is particularly significant for applications requiring robust and energy-efficient solutions, such as environmental monitoring and healthcare systems. The framework establishes a foundation for integrating advanced techniques like reinforcement learning and multi-objective optimization, advancing the field of energy-efficient WSN design and its diverse real-world applications.
Summary of the Study:
This study introduces an AI-driven adaptive clustering framework for Wireless Sensor Networks (WSNs) that enhances energy efficiency and extends network lifespan. By integrating machine learning with bio-inspired algorithms like Salp Swarm Optimization (SSO) and Genetic Algorithm (GA), the framework enables dynamic Cluster Head (CH) selection based on real-time network conditions. Compared to traditional protocols such as LEACH and HEED, the method reduces energy consumption by 30%, increases network lifetime by 25%, and improves data throughput by 20%, demonstrating its suitability for energy-critical applications like smart cities and healthcare.