Arthy P. S., Chandra Sekar P., Praveenkumar Babu
1 Department of Electronics and Communication Engineering, Sri Sai Ram Institute of Technology, West Tambaram, Chennai, India.
2 Department of Electronics and Communication Engineering, Siddartha Institute of Science and Technology, Puttur-517583, Andhra Pradesh, India.
3 Department of Electronics and Communication Engineering, SRM Institute of Science and Technology, Ramapuram, Chennai-600089, India.
* Author to whom correspondence should be addressed:
mbp.praveen@gmail.com (P. Babu)
ABSTRACT
Human-AI collaboration is rapidly advancing due to breakthroughs in multimodal interaction technologies, enabling intuitive communication across speech, vision, gestures, and text. This study investigates emerging paradigms that enhance Human-AI collaboration by integrating multimodal frameworks, which foster seamless, dynamic, and context-aware interactions. Leveraging advancements in artificial intelligence, including natural language processing, computer vision, and sensory data fusion, the proposed frameworks align closely with human cognitive processes, enabling mutual understanding and improved task efficiency. One key focus of this research is addressing critical challenges such as context comprehension, adaptability to diverse user needs, and ethical considerations surrounding AI integration. The study explores novel strategies to improve system responsiveness, including attention-based models for task prioritization, real-time synchronization techniques, and reinforcement learning approaches. Additionally, privacy-preserving mechanisms and bias mitigation strategies are incorporated to ensure secure and inclusive operation. Experimental validations demonstrate significant improvements in user satisfaction, response accuracy, and communication efficiency when compared to unimodal systems. The study highlights the transformative potential of multimodal frameworks in domains such as healthcare, education, and smart environments, where dynamic collaboration and decision-making are paramount. By providing a comprehensive perspective on the design principles, evaluation metrics, and domain-specific applications, this research underscores the importance of multimodal interaction systems in redefining Human-AI partnerships. Overall, the study positions multimodal interaction as a foundational element for enhancing AI’s role in collaborative problem-solving, paving the way for more natural, ethical, and scalable Human-AI interaction systems across diverse applications.
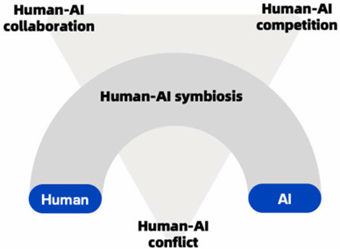
Significance of the Study:
This study highlights the transformative potential of multimodal interaction frameworks in advancing Human-AI collaboration. By integrating speech, vision, gestures, and text, it addresses critical challenges like context comprehension, adaptability, and ethical AI operation. The research demonstrates improved accuracy, user satisfaction, and system responsiveness, ensuring secure, inclusive, and scalable AI solutions. Its applicability in domains such as healthcare, education, and smart environments positions multimodal frameworks as foundational for enhancing intuitive and effective Human-AI partnerships.
Summary of the Study:
This research presents a novel multimodal interaction framework for enhancing Human-AI collaboration. Integrating sensory data fusion, context-aware processing, and adaptive learning, the framework facilitates dynamic and intuitive communication across diverse modalities. Experimental validation revealed significant improvements in task efficiency, accuracy, and user satisfaction compared to unimodal systems. Key contributions include reinforcement learning for decision-making, privacy-preserving mechanisms, and bias mitigation strategies. The study emphasizes its application in healthcare, education, and smart environments, establishing a foundation for natural, ethical, and scalable Human-AI systems.