Susani Antony Mrefu, Zubaer Ibna Mannan, Nur Alam MD
Department of Smart Computing, Kyungdong University (Global Campus), Gangwon-do, Republic of Korea.
* Author to whom correspondence should be addressed:
na@kduniv.ac.kr (Nur Alam MD)
ABSTRACT
Plant phenotyping has become a pivotal aspect of modern agriculture, providing tools for monitoring plant health and improving crop yield. This study introduces an efficient and cost-effective image processing approach for detecting and segmenting healthy and diseased plant leaves. The methodology focuses on three primary stages: preprocessing, segmentation, and post-processing. During preprocessing, the acquired leaf images are enhanced using grayscale conversion to eliminate noise and simplify image analysis. In the segmentation phase, thresholding techniques are applied to isolate the leaf from its background, enabling more accurate disease identification. The post-processing stage involves refining the segmented leaf images, detecting edges, and analyzing contours to better understand the shape and structure of the leaves. To evaluate the proposed method, a dataset consisting of 100 leaf images was utilized. Experimental results demonstrated that the algorithm successfully detected 68% of infected leaves and 75% of healthy leaves, achieving an overall accuracy of 66.66%. These findings highlight the potential of grayscale conversion and thresholding techniques in automating disease detection, particularly in resource-limited agricultural environments. While the method provides promising results, limitations such as dataset diversity and reliance on grayscale processing were identified. Future enhancements will include the incorporation of color information, the application of advanced machine learning algorithms, and the use of larger, more diverse datasets to improve accuracy and robustness. Overall, this study presents a foundational step toward the development of automated systems for efficient plant disease monitoring and management in precision agriculture.
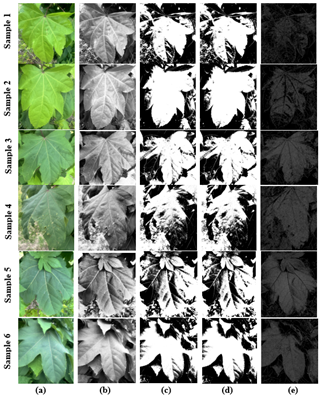
Significance of the Study:
This study provides an efficient, cost-effective image processing method for detecting and segmenting plant leaf diseases, particularly valuable in resource-limited agricultural settings. By automating disease detection, the approach aids farmers in identifying issues early, improving crop management and productivity. The integration of grayscale conversion and thresholding simplifies the process, making it accessible and scalable for real-world applications. Future enhancements, including color analysis and machine learning, can further strengthen its impact on precision agriculture.
Summary of the Study:
The study introduces an image-based method for detecting and segmenting plant leaf diseases through three stages: preprocessing, segmentation, and post-processing. Grayscale conversion simplifies image analysis, while thresholding isolates leaves from backgrounds for accurate disease detection. Experimental evaluation on 100 images achieved 66.66% accuracy, identifying 68% of diseased and 75% of healthy leaves. The method demonstrates potential for real-world agricultural applications, though limitations such as dataset diversity and omission of color data highlight opportunities for future improvements.