Ashish Thapa, Sami Azam, Nur Alam MD, Zubaer Ibna Mannan
1 Department of Smart Computing, Kyungdong University (Global Campus), Gangwon-do, Republic of Korea.
2 College of Engineering, IT and Environment, Charles Darwin University, NT, Australia.
* Author to whom correspondence should be addressed:
zimannan@kduniv.ac.kr (Z. I. Mannan)
ABSTRACT
Breast cancer, particularly Invasive Ductal Carcinoma (IDC), remains one of the most prevalent and life-threatening cancers affecting women globally. Early and accurate detection is critical for improving treatment outcomes, making automated classification systems increasingly vital in healthcare. This study proposes an automated breast cancer classification system that integrates Support Vector Machine (SVM) and Convolutional Neural Network (CNN) classifiers, utilizing features such as data patches and color variations extracted from histopathological images. The proposed system focuses on accurately classifying breast cancer histopathological images into benign or malignant categories. The SVM classifier demonstrates superior performance, outperforming CNN and traditional classifiers such as Decision Tree, Naïve Bayes, and Nearest Neighbors in terms of accuracy, sensitivity, and specificity. It also surpasses CNN in key metrics, including precision, recall, and F1-score, establishing its robustness and reliability in image classification tasks. The study evaluates the proposed SVM architecture on the IDC dataset, achieving an accuracy of 94%. This result underscores the potential of the SVM classifier for developing computer-assisted diagnostic (CAD) systems, which can enhance the efficiency and accuracy of breast cancer diagnosis. Furthermore, the system demonstrates the ability to localize cancerous tissues within whole histopathological images, offering a comprehensive diagnostic tool for pathologists. This study highlights the effectiveness of integrating machine learning models for breast cancer classification and emphasizes the importance of automated systems in augmenting diagnostic accuracy, reducing workload, and advancing personalized cancer care.
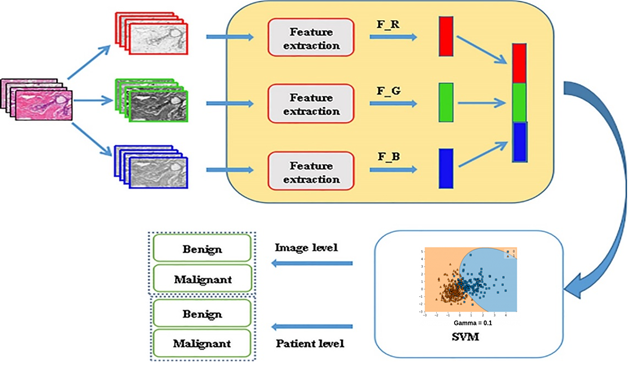
Significance of the Study:
This study introduces an integrated SVM-CNN framework to improve the accuracy and reliability of breast cancer classification from histopathological images. By achieving a 94% accuracy on the IDC dataset, the SVM model outperforms traditional classifiers and CNNs, emphasizing its robustness and precision. The ability to localize cancerous tissues enhances its value as a diagnostic aid, reducing workload for pathologists while improving diagnostic speed and consistency. This research paves the way for scalable, efficient, and accurate AI-driven cancer diagnostic solutions.
Summary of the Study:
This research presents an automated breast cancer classification system that integrates SVM and CNN frameworks to classify histopathological images into benign and malignant categories. The SVM classifier outperforms CNN and traditional methods, achieving 94% accuracy on the IDC dataset. It excels in precision, recall, and F1-score, demonstrating its effectiveness in localizing cancerous tissues. The study highlights the system’s potential as a reliable computer-assisted diagnostic tool, emphasizing its role in enhancing diagnostic accuracy, reducing pathologist workload, and supporting personalized cancer care.