S. Prabu, R. Uma Maheshwari, K. Kalpana, K. Mahendrakan
1 Department of Electronics and Communication Engineering, Mahendra Engineering College, Mallasamudram, Namakkal DT, Tamilnadu, India.
2 Department of Electronics and Communication Engineering, Hindusthan Institute of Technology, Coimbatore-641032, Tamilnadu, India.
* Author to whom correspondence should be addressed:
vsprabu4u@gmail.com (S. Prabu)
ABSTRACT
This study introduces an innovative Adaptive Learning Path Optimization Algorithm (ALPOA) designed to enhance personalized learning in e-learning environments. The algorithm employs a combination of machine learning techniques and rule-based systems to dynamically adjust and customize learning pathways based on individual student performance, preferences, and behavior. By analyzing real-time data, the algorithm tailors content delivery, recommends relevant resources, and aligns learning activities with evolving knowledge levels. Experimental results reveal notable improvements, including a 15% average increase in test scores, a 25% rise in learner engagement, and a 25% reduction in dropout rates. These outcomes underscore the effectiveness of ALPOA in creating adaptive, efficient, and engaging learning experiences. The study further explores the scalability of the algorithm, demonstrating its applicability across diverse educational contexts such as K-12, higher education, and corporate training. By leveraging real-time analytics and predictive modeling, ALPOA provides a robust framework for addressing the challenges of individualizing education at scale. The proposed system not only optimizes learning outcomes but also promotes user satisfaction by fostering an engaging and personalized learning journey. The findings highlight the transformative potential of adaptive algorithms in e-learning, paving the way for a more inclusive and effective digital education ecosystem. Future research aims to refine ALPOA’s architecture, test its efficacy in various real-world settings, and address challenges related to data security and scalability.
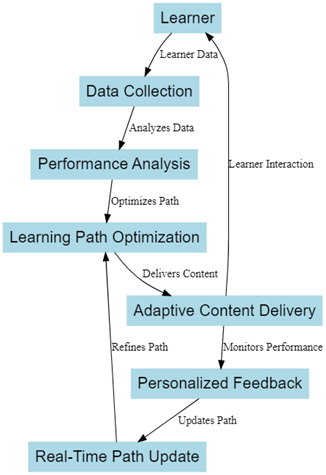
Significance of the Study:
This study introduces the Adaptive Learning Path Optimization Algorithm (ALPOA) to revolutionize personalized e-learning. By dynamically tailoring educational pathways to individual learner preferences and performance in real-time, ALPOA enhances engagement, improves outcomes, and reduces dropout rates. Its scalability across diverse educational contexts makes it a transformative tool for addressing the global challenge of individualizing education. This innovation promotes inclusivity, efficiency, and satisfaction, paving the way for more effective and adaptable e-learning systems worldwide.
Summary of the Study:
The Adaptive Learning Path Optimization Algorithm (ALPOA) dynamically customizes e-learning pathways using machine learning and rule-based techniques. Experimental findings show a 15% test score improvement, a 25% rise in engagement, and a 25% reduction in dropout rates. ALPOA’s scalability is demonstrated across K-12, higher education, and corporate training, offering a robust framework for personalized education. The study highlights its potential to optimize learning outcomes and foster learner satisfaction while addressing scalability and data security challenges in future developments.