A. S. Y. Bin-Habtoor, Kainat Fatima
1 Department of Computer Network & Communication, Collage of Engineering, Hadramout University, Yemen.
2 Department of Computer Science, Magadh University, Bodh Gaya, Bihar, India
* Author to whom correspondence should be addressed:
drazizhabtoor@gmail.com (A. S. Y. Bin-Habtoor)
ABSTRACT
Moreover, as organizations implement robust predictive maintenance strategies, they can leverage artificial intelligence (AI) to minimize human intervention in data analysis, leading to more automated and self-sustaining maintenance systems. This progress has been demonstrated by using machine learning methods such as critical decision trees and neural networks to analyze operational data and predict equipment failure. The validation of machine learning models against actual operational data enhances the reliability of these predictive maintenance systems, providing a strong foundation for their broader application. The development of condition monitoring systems for industrial equipment is driven by advances in data transmission, storage technologies, and decreasing costs of reliable sensors. Also, Internet of Things (IoT) enables instant exchange of detailed data collected from various monitoring devices. This partnership creates a valuable opportunity for predictive maintenance by integrating efficient data collection with advanced analytics. For example, image-based predictive maintenance using drone camera surveys for structural monitoring is gaining popularity in various industries. The results indicate that Model E achieved the highest rank, while Model D had the lowest rank being attained. The value of the dataset Using AI for Predictive Maintenance, according to the MOORA method, Model E achieves the highest ranking.
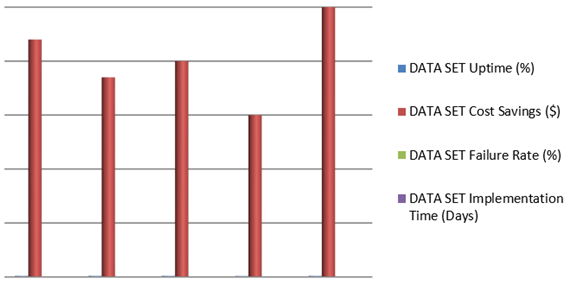
Significance of the Study:
The integration of IoT and AI in predictive maintenance revolutionizes industrial operations by enabling proactive strategies that reduce costs, downtime, and failures. By automating data analysis, these systems enhance operational efficiency and asset reliability. Techniques like image-based drone monitoring and AI-driven models improve situational awareness, making industries safer and more cost-effective. The MOORA method’s application underscores its utility in optimizing decision-making, paving the way for widespread adoption of advanced predictive maintenance solutions.
Summary of the Study:
This study explores the integration of IoT and AI for advanced predictive maintenance systems, employing the MOORA method to evaluate various condition monitoring models. By leveraging real-time data exchange from IoT devices and AI-driven analytics, the study demonstrates the shift from reactive to proactive maintenance strategies. Image-based drone surveys and machine learning models, such as decision trees and neural networks, enhance failure prediction accuracy. Model E ranks highest, showcasing its effectiveness in predictive maintenance for industrial equipment.